Predicting overactive bladder from inflammatory markers: A machine learning approach using NHANES 2005–2020
DOI:
https://doi.org/10.17305/bb.2025.12335Keywords:
Overactive bladder, OAB, inflammatory biomarkers, machine learning, National Health and Nutrition Examination Survey, NHANES, predictive modelingAbstract
Overactive bladder (OAB), a prevalent condition characterized by urgency and nocturia, imposes significant burdens on both quality of life and healthcare systems. Emerging evidence implicates systemic inflammation in OAB pathogenesis; however, the role of complete blood count (CBC)-derived inflammatory biomarkers remains underexplored. This cross-sectional study analyzed data from 35,394 participants in the National Health and Nutrition Examination Survey (NHANES, 2005–2020) to evaluate associations between CBC-derived biomarkers—such as the Systemic Immune-Inflammation Index (SII), Systemic Inflammation Response Index (SIRI), and Neutrophil-to-Lymphocyte Ratio (NLR)—and OAB (defined by an OAB Symptom Score ≥3). Multivariable logistic regression, threshold analysis, and machine learning models (Random Forest [RF], Extreme Gradient Boosting) were employed, adjusting for sociodemographic, lifestyle, and clinical covariates. Elevated levels of SII, SIRI, NLR, Monocyte-to-Lymphocyte Ratio (MLR), and Neutrophil-MLR (NMLR) were significantly associated with increased OAB risk (all P < 0.05), with adjusted odds ratios for the highest quartiles ranging from 1.21 (SII; 95% CI: 1.10–1.34) to 1.31 (NMLR; 1.19–1.44). Nonlinear associations were observed, with inflection points (e.g., NLR = 1.071, MLR = 0.174) marking abrupt increases in risk. RF models showed strong predictive performance (area under the curve = 0.89 for training; 0.76 for testing), identifying SII and SIRI as key predictors. Subgroup analyses demonstrated consistent associations across most demographic groups, with the exception of hyperlipidemia, which modified the effects of SIRI, NLR, and NMLR. These findings highlight the role of systemic inflammation in OAB and suggest that CBC-derived biomarkers could serve as cost-effective tools for risk stratification. The integration of epidemiological analysis and machine learning enhances our understanding of OAB’s inflammatory underpinnings, although longitudinal studies are needed to establish causal relationships and therapeutic implications.
Citations
Downloads
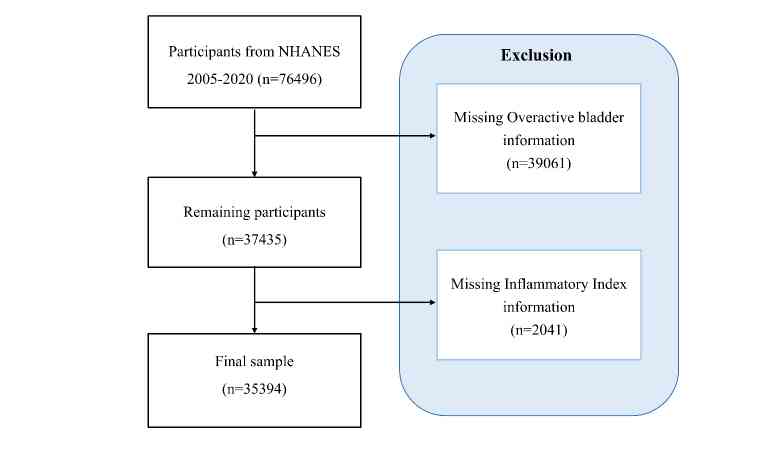
Downloads
Published
Issue
Section
Categories
License
Copyright (c) 2025 Haoxun Zhang, Guoling Zhang, Chunyang Wang

This work is licensed under a Creative Commons Attribution 4.0 International License.