In vitro toxicity model: Upgrades to bridge the gap between preclinical and clinical research
DOI:
https://doi.org/10.17305/bjbms.2019.4378Keywords:
Toxicity models, cell-based models, in vitro, physiological models, bioanalysis, drug approval, toxicity testing, toxicity prediction, drug toxicity, preclinical researchAbstract
The Centers for Disease Control and Prevention (CDC) provides extensive data that indicate our need for drugs to maintain human population health. Despite the substantial availability of drugs on the market, many patients lack specific drugs. New drugs are required to tackle this issue. Moreover, we need more reliable models for testing drug toxicity, as too many drug approval failures occur with the current models. This article briefly describes various approaches of the currently used models for toxicity screening, to justify the selection of in vitro cell-based models. Cell-based toxicity models have the best potential to reliably predict drug toxicity in humans, as they are developed using the cells of the target organism. However, currently, a large gap exists between in vitro cell-based approach to toxicity testing and the clinical approach, which may be contributing to drug approval failures. We propose improvements to in vitro cell-based toxicity models, which is often an insight approach, to better match this approach with the clinical homeostatic approach. This should enable a more accurate comparison of data between the preclinical as well as clinical models and provide a more comprehensive understanding of human physiology and biological effects of drugs.
Citations
Downloads
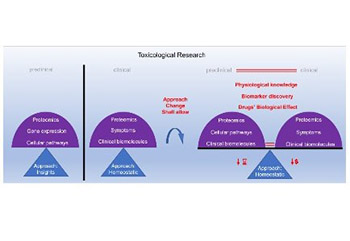
Downloads
Additional Files
Published
How to Cite
Accepted 2019-09-29
Published 2020-05-01