Computed tomography in the diagnosis of intraperitoneal effusions: The role of texture analysis
DOI:
https://doi.org/10.17305/bjbms.2020.5048Keywords:
Ascites, computed tomography, CT, diagnostic-aid, image processing, texture-based analysisAbstract
The morphological changes advocating for peritoneal carcinomatosis are inconsistent and may be visible only in later stages of the disease. However, malignant ascites represents an early sign, and this fluid exhibits specific histological characteristics. This study aimed to quantify the fluid properties on computed tomography (CT) images of intraperitoneal effusions through texture analysis and evaluate its utility in differentiating benign and malignant collections. Fifty-two patients with histologically proven benign (n=29) and malignant (n=23) intraperitoneal effusions who underwent CT examinations were retrospectively included. Texture analysis of the fluid component was performed on the non-enhanced phase of each examination using dedicated software. Fisher and the probability of classification error and average correlation coefficients were used to select two sets of ten texture features, whose ability to distinguish between the two types of collections were tested using a k-nearest-neighbor classifier. Also, each of the selected feature’s diagnostic power was assessed using univariate and receiver operating characteristics analysis with the calculation of the area under the curve. The k-nearest-neighbor classifier was able to distinguish between the two entities with 71.15% accuracy, 73.91% sensitivity, and 68.97% specificity. The highest-ranked texture parameter was Inverse Difference Moment (p=0.0023; area under the curve=0.748), based on which malignant collections could be diagnosed with 95.65% sensitivity and 44.83% specificity. Although successful, the texture assessment of benign and malignant collections most likely does not reflect the cytological differences between the two groups.
Citations
Downloads
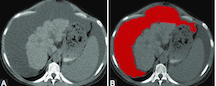