Bioinformatics analysis reveals TSPAN1 as a candidate biomarker of progression and prognosis in pancreatic cancer
DOI:
https://doi.org/10.17305/bjbms.2020.5096Keywords:
Pancreatic Cancer, TSPAN1, TCGA, GEO dataset, Diagnosis and prognosis, KEGGAbstract
Pancreatic cancer (PCC) is a common malignant tumor of the digestive system that is resistant to traditional treatments and has an overall 5-year survival rate of <7%. Transcriptomics research provides reliable biomarkers for diagnosis, prognosis, and clinical precision treatment, as well as the identification of molecular targets for the development of drugs to improve patient survival. We sought to identify new biomarkers for PCC by combining transcriptomics and clinical data with current knowledge regarding molecular mechanisms. Consequently, we employed weighted gene co-expression network analysis and differentially expressed gene analysis to evaluate genes co-expressed in tumor versus normal tissues using pancreatic adenocarcinoma data from The Cancer Genome Atlas and dataset GSE16515 from the Gene Expression Omnibus. Twenty-one overlapping genes were identified, with enrichment of key Gene Ontology and Kyoto Encyclopedia of Genes and Genomes pathways, including epidermal growth factor receptor signaling, cadherin, cell adhesion, ubiquinone, and glycosphingolipid biosynthesis pathways, and retinol metabolism. Protein-protein interaction analysis highlighted 10 hub genes, according to Maximal Clique Centrality. Univariate and multivariate COX analyses indicated that TSPAN1 serves as an independent prognostic factor for PCC patients. Survival analysis distinguished TSPAN1 as an independent prognostic factor among hub genes in PCC. Finally, immunohistochemical staining results suggested that the TSPAN1 protein levels in the Human Protein Atlas were significantly higher in tumor tissue than in normal tissue. Therefore, TSPAN1 may be involved in PCC development and act as a critical biomarker for diagnosing and predicting PCC patient survival.
Citations
Downloads
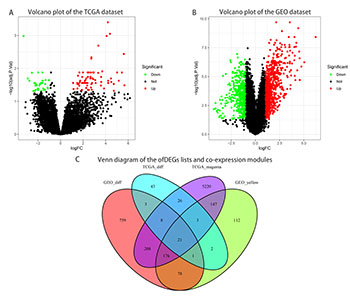
Downloads
Additional Files
Published
Issue
Section
Categories
How to Cite
Accepted 2020-11-08
Published 2021-02-01