Machine learning as the new approach in understanding biomarkers of suicidal behavior
DOI:
https://doi.org/10.17305/bjbms.2020.5146Keywords:
Suicide, artificial intelligence, personalized medicine, precision medicine, precision psychiatryAbstract
In psychiatry, compared to other medical fields, the identification of biological markers that would complement current clinical interview, and enable more objective and faster clinical diagnosis, implement accurate monitoring of treatment response and remission, is grave. Current technological development enables analyses of various biological marks in high throughput scale at reasonable costs, and therefore ‘omic’ studies are entering the psychiatry research. However, big data demands a whole new plethora of skills in data processing, before clinically useful information can be extracted. So far the classical approach to data analysis did not really contribute to identification of biomarkers in psychiatry, but the extensive amounts of data might get to a higher level, if artificial intelligence in the shape of machine learning algorithms would be applied. Not many studies on machine learning in psychiatry have been published, but we can already see from that handful of studies that the potential to build a screening portfolio of biomarkers for different psychopathologies, including suicide, exists.
Citations
Downloads
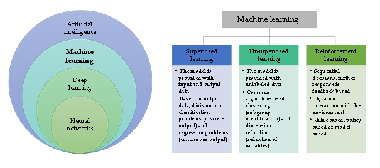
Downloads
Additional Files
Published
How to Cite
Accepted 2020-12-15
Published 2021-08-01