Construction and validation of a preterm birth risk assessment model using fuzzy analytic hierarchy process
DOI:
https://doi.org/10.17305/bjbms.2021.6431Keywords:
Preterm birth, fuzzy multi-criteria analysis, fuzzification, risk assessment, decision makingAbstract
Preterm births account for almost 1 million deaths globally. The objective of this study is to develop and evaluate a model that assists clinicians in assessing the risk of preterm birth, using fuzzy multicriteria analysis. The model allows experts to incorporate their intuition and judgment into the decision-making process and takes into consideration six (6) risk dimensions reflecting the socio-economic, behavioural and medical profile of pregnant women, thus adopting a holistic approach to risk assessment. Each risk dimension is further analysed and measured in terms of risk factors associated with it. Data was collected from a selected group of 35 experts, each one with more than 20 years of obstetric experience. The model criteria were selected after a thorough literature analysis, so as to ensure a holistic approach to risk assessment. The criteria were reviewed by the experts and the model structure was finalised. The fuzzy analytic hierarchy method was applied to calculate the relative importance of each criterion and subsequent use of the model in assessing and ranking pregnant women by their preterm risk. The proposed model utilises fuzzy logic and multicriteria analysis. It addresses the multifactorial nature of decision making when assessing the preterm birth risk. It also incorporates the obstetricians’ intuitive judgement during risk assessment and it can be used to classify cases based upon their risk level. Additionally, it can be applied to evaluate the risk of individual cases in a personalised manner. The proposed model is compared and validated for its predictive value against judgments made by experts.
Citations
Downloads
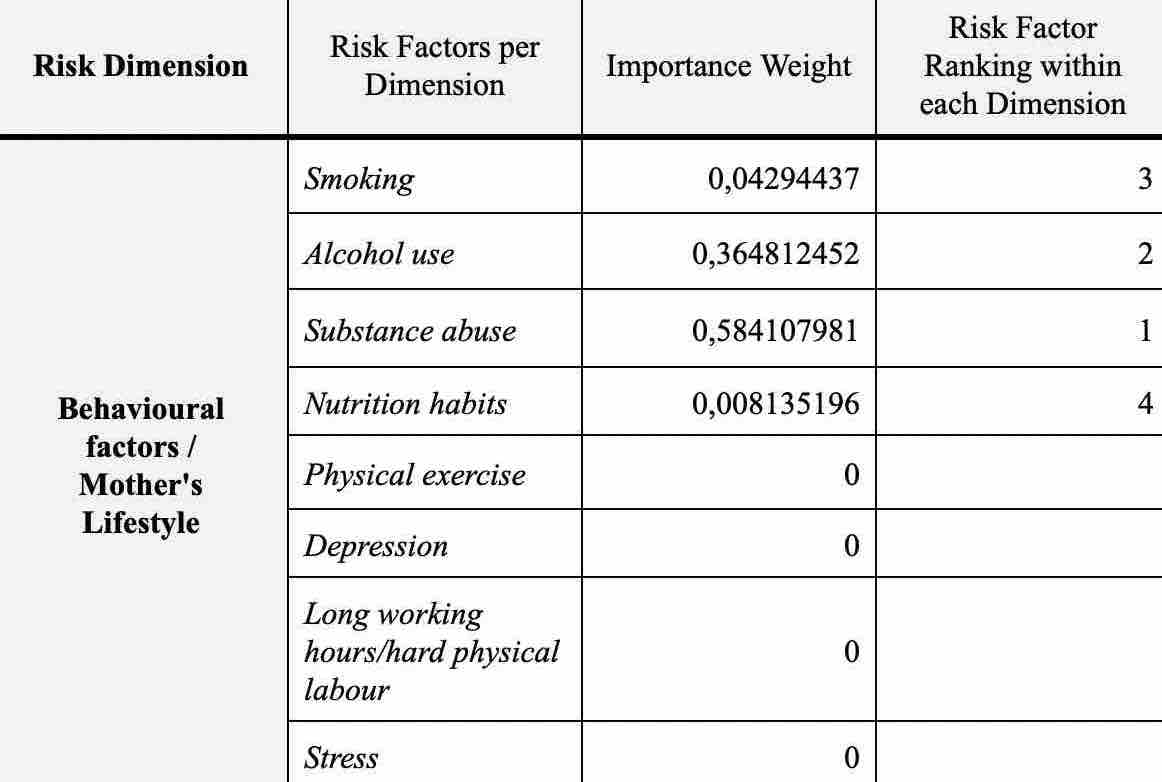