An improved joint non-negative matrix factorization for identifying surgical treatment timing of neonatal necrotizing enterocolitis
DOI:
https://doi.org/10.17305/bjbms.2022.7046Keywords:
Neonatal necrotizing enterocolitis, joint non-negative matrix factorization, surgical indications, multimodal clinical dataAbstract
Neonatal necrotizing enterocolitis is a severe neonatal intestinal disease. Timely identification of surgical indications is essential for newborns in order to seek the best time for treatment and improve prognosis. This paper attempts to establish an algorithm model based on multimodal clinical data to determine the features of surgical indications and construct an auxiliary diagnosis model. The proposed algorithm adds hypergraph constraints on the two modal data based on Joint Nonnegative Matrix Factorization (JNMF), aiming to mine the higher-order correlations of the two data features. In addition, the adjacency matrix of the two kinds of data is used as a network regularization constraint to prevent overfitting. Orthogonal and L1-norm regulations were introduced to avoid feature redundancy and perform feature selection, respectively, and confirmed 14 clinical features. Finally, we used three classifiers, random forest, support vector machine, and logistic regression, to perform binary classification of patients requiring surgery. The results show that when the features selected by the proposed algorithm model are classified by random forest, the area under the ROC curve is 0.8, which has high prediction accuracy.
Citations
Downloads
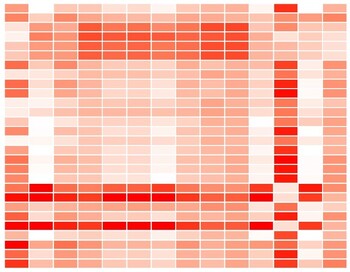
Downloads
Additional Files
Published
Issue
Section
Categories
License
Copyright (c) 2022 Guoqiang Qi, Shoujiang Huang, Dengming Lai, Jing Li, Yonggen Zhao, Chen Shen, Jian Huang, Tianmei Liu, Kai Wei, Jinfa Dou, Qiang Shu, Gang Yu

This work is licensed under a Creative Commons Attribution 4.0 International License.
How to Cite
Accepted 2022-05-04
Published 2022-10-23