Prediction of pathologic complete response prediction in patients with locally advanced esophageal squamous cell carcinoma treated with neoadjuvant immunochemotherapy: A real-world study
DOI:
https://doi.org/10.17305/bjbms.2022.7696Keywords:
Esophageal squamous cell carcinoma, systemic immune-inflammation index, neutrophil lymphocyte ratio, prognostic nutritional index, lymphocyte monocyte ratio, platelet lymphocyte ratio, pathologic complete responseAbstract
As an emerging hotspot for patients with locally advanced esophageal squamous cell carcinoma (LA-ESCC), neoadjuvant immunochemotherapy (nICT) is safe and feasible. Pathological complete response (pCR) is considered to be an important therapeutic effect of neoadjuvant therapy. However, few studies have explored pCR predictors for nICT in LA-ESCC. The purpose of this study was to predict pCR after nICT in LA-ESCC by pretreatment clinical characteristics and hematological indexes. The primary endpoint was to explore the impacts on the predictors for pCR prediction. Clinical characteristics and hematological indexes including systemic immune-inflammation index (SII), neutrophil lymphocyte ratio (NLR), lymphocyte monocyte ratio (LMR), prognostic nutritional index (PNI) and platelet lymphocyte ratio (PLR) were conducted. A total of 150 LA-ESCC patients were enrolled in the current study. There were 14 (9.3%) female and 136 (90.7%) male patients. Fifty-two patients achieved pCR (34.7%). A higher pCR rate was found in low-NLR group (43.7% vs. 26.6%, P=0.028) and high-LMR group (43.8% vs. 21.3%, P=0.004), respectively. Differentiation [odds ratio (OR) =0.464, 95% confidence interval (CI)=0.259-0.830, P=0.010], LMR (OR=0.309, 95% CI=0.132-0.707, P=0.007) and cTNM (OR=0.225, 95% CI=0.115-0.441, P<0.001) were independent predictors for pCR. The nomogram for pCR prediction based on LMR, differentiation and cTNM stage had good discrimination performance and calibration coordination (C-index=0.779). The results of our study are of great significance for designing therapeutic strategies. Nomogram based on LMR, differentiation and cTNM may accurately and effectively predict pCR.
Downloads
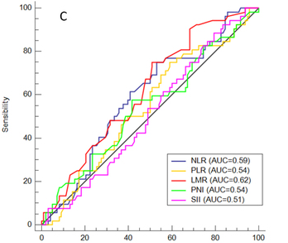
Downloads
Additional Files
Published
Issue
Section
Categories
License
Copyright (c) 2022 Jifeng Feng, Liang Wang, Xun Yang, Qixun Chen, Xiangdong Cheng

This work is licensed under a Creative Commons Attribution 4.0 International License.