Machine learning to improve prognosis prediction of metastatic clear-cell renal cell carcinoma treated with cytoreductive nephrectomy and systemic therapy
DOI:
https://doi.org/10.17305/bjbms.2022.8047Keywords:
Metastatic clear-cell renal cell carcinoma, cytoreductive nephrectomy, systemic therapy, machine learning, prognosis prediction model, SEER databaseAbstract
Cytoreductive nephrectomy (CN) combined with systemic therapy is commonly used to treat metastatic clear-cell renal cell carcinoma (mccRCC). However, prognostic models for these patients are limited. In the present study, the clinical data of 782 mccRCC patients who received both CN and systemic therapy were obtained from the Surveillance, Epidemiology, and End Results (SEER) database (2010-2016), and patients were divided into training and internal test cohorts. A total of 144 patients who met the same criteria from our center (Peking Union Medical College Hospital) were placed in the external test cohort. The cancer-specific survival rate (CSS) at 1, 3, and 5 years was set as the research outcome. Then, four ML models, i.e., a gradient boosting machine (GBM), support vector machine (SVM), random forest (RF), and logistic regression (LR), were established. Fifteen potential independent features were included in this study. Model performance was evaluated using the area under the receiver operating characteristic curves (AUC), calibration plots, and decision curve analysis (DCA). Seven clinical features, namely pathological grade, T stage, N stage, number of metastatic sites, brain or liver metastases, and metastasectomy were selected for subsequent analysis via the recursive feature elimination (RFE) algorithm. In conclusion, the GBM model performed best at 1-, 3- and 5-year CSS prediction (0.836, 0.819 and 0.808, respectively in the internal test cohort and 0.819, 0.805 and 0.786, respectively in the external cohort). Furthermore, we divided the patients into three strata (high-, intermediate- and low-risk) via X-tile analysis and concluded that clinically individualized treatment can be aided by these practical prognostic models.
Citations
Downloads
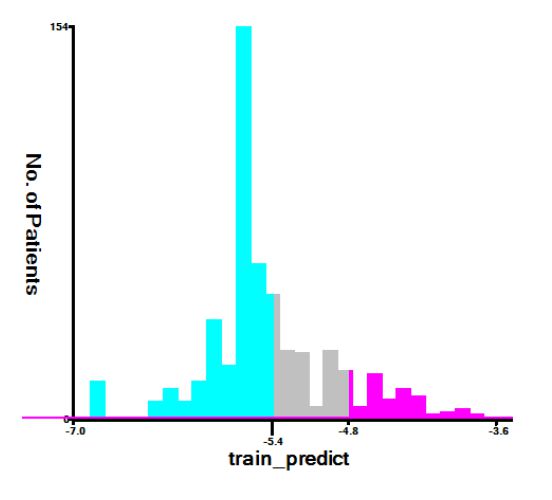
Downloads
Additional Files
Published
Issue
Section
Categories
License
Copyright (c) 2022 Wenjie Yang, Lin Ma, Jie Dong, Mengchao Wei, Ruoyu Ji, Hualin Chen, Xiaoqiang Xue, YIngjie Li, Zhaoheng Jin, Weifeng Xu, Zhigang Ji

This work is licensed under a Creative Commons Attribution 4.0 International License.