Electrodermal activity and heart rate variability for detection of peripheral abnormalities in type 2 diabetes: A review
DOI:
https://doi.org/10.17305/bb.2022.8561Keywords:
Diabetes mellitus, diabetic foot ulcer, autonomic neuropathy, electrodermal activity, heart rate variabilityAbstract
Modern medicine exhibits an upward trend towards non-invasive methods for early detection of disease and long-term monitoring of patients’ health. Diabetes mellitus and its complications are a promising area for implementation of new medical diagnostic devices. One of the most serious complications of diabetes is diabetic foot ulcer. The main causes responsible for diabetic foot ulcer are ischemia caused by peripheral artery disease and diabetic neuropathy caused by polyol pathway-induced oxidative stress. Autonomic neuropathy impairs function of sweat glands, which can be measured by electrodermal activity. On the other hand, autonomic neuropathy leads to changes in heart rate variability, which is used to assess autonomic regulation of the sinoatrial node. Both methods are enough sensitive to detect pathological changes caused by autonomic neuropathy and are promising screening methods for early diagnosis of diabetic neuropathy, which could prevent the onset of diabetic ulcer.
Citations
Downloads
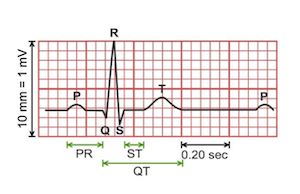
Downloads
Additional Files
Published
License
Copyright (c) 2023 Matej Žnidarič, Dominik Škrinjar, Alen Kapel

This work is licensed under a Creative Commons Attribution 4.0 International License.
How to Cite
Accepted 2023-02-12
Published 2023-09-04