Identification of new biomarkers and immune infiltration characteristics of sepsis in very low birth weight infants
DOI:
https://doi.org/10.17305/bb.2023.8966Keywords:
Sepsis, very low birth weight (VLBW) infants, weighted gene co-expression network analysis (WGCNA), immune infiltration, biomarkerAbstract
Sepsis is a life-threatening condition, especially in very low birth weight (VLBW) infants, and its pathogenesis remains unclear. Effective biomarkers need to be found to diagnose and treat the disease at an early stage. The Gene Expression Omnibus (GEO) database was screened and analyzed for differentially expressed genes (DEGs) in VLBW infants with sepsis. DEGs were then analyzed for functional enrichment. A weighted gene co-expression network analysis (WCGNA) was performed to identify the key modules and genes. The optimal feature genes (OFGs) were created using three machine learning algorithms. The single-sample Gene Set Enrichment Analysis (ssGSEA) scored the degree of immune cell enrichment between septic and control patients, and the correlation between OFGs and immune cells was evaluated. A total of 101 DEGs were identified between the sepsis and control samples. DEGs were mainly associated with immune responses and inflammatory signaling pathways in the enrichment analysis. In the WGCNA analysis, the MEturquoise module was significantly correlated with sepsis in VLBW infants (cor = 0.57, P < 0.001). By intersecting OFGs derived from three machine learning algorithms, two biomarkers were identified: glycogenin 1 (GYG1) and resistin (RETN). The area under the curves of GYG1 and RETN was greater than 0.97 in the testing set. The ssGSEA indicated immune cells infiltration in septic VLBW infants, and GYG1 and RETN revealed close correlations with immune cells. New biomarkers offer promising insights into the diagnosis and treatment of sepsis in VLBW infants.
Citations
Downloads
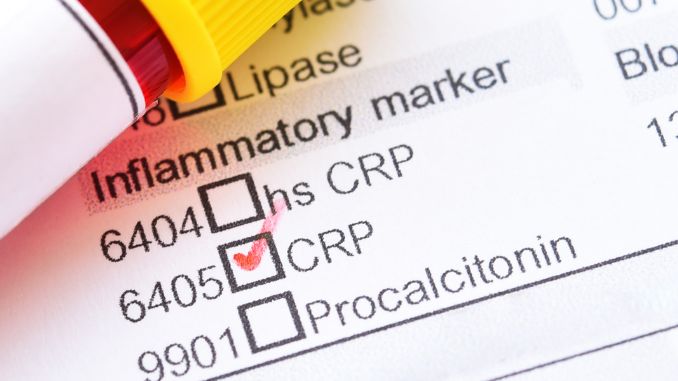
Downloads
Additional Files
Published
Issue
Section
Categories
License
Copyright (c) 2023 Yujia Luo, Zhou Jiang, Rei Gu, Xuandong Zhang, Li Wei, Yuanyuan Zhou, Songying Zhang

This work is licensed under a Creative Commons Attribution 4.0 International License.
How to Cite
Accepted 2023-04-23
Published 2023-09-04