Leveraging artificial intelligence to identify high-risk patients for postoperative sore throat: An observational study
DOI:
https://doi.org/10.17305/bb.2023.9519Keywords:
Random forest (RF), neural network (NN), extreme gradient boosting (XGBoost), postoperative sore throat (POST)Abstract
Postoperative sore throat (POST) is a prevalent complication after general anesthesia and targeting high-risk patients helps in its prevention. This study developed and validated a machine learning model to predict POST. A total number of 834 patients who underwent general anesthesia with endotracheal intubation were included in this study. Data from a cohort of 685 patients was used for model development and validation, while a cohort of 149 patients served for external validation. The prediction performance of random forest (RF), neural network (NN), and extreme gradient boosting (XGBoost) models was compared using comprehensive performance metrics. The Local Interpretable Model-Agnostic Explanations (LIME) methods elucidated the best-performing model. POST incidences across training, validation, and testing cohorts were 41.7%, 38.4%, and 36.2%, respectively. Five predictors were age, sex, endotracheal tube cuff pressure, endotracheal tube insertion depth, and the time interval between extubation and the first drinking of water after extubation. After incorporating these variables, the NN model demonstrated superior generalization capabilities in predicting POST when compared to the XGBoost and RF models in external validation, achieving an area under the receiver operating characteristic curve (AUROC) of 0.81 (95% CI 0.74–0.89) and a precision–recall curve (AUPRC) of 0.77 (95% CI 0.66–0.86). The model also showed good calibration and clinical usage values. The NN model outperforms the XGBoost and RF models in predicting POST, with potential applications in the healthcare industry for reducing the incidence of this common postoperative complication.
Citations
Downloads
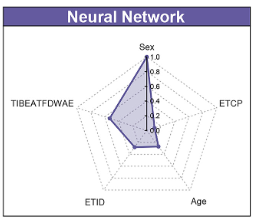
Downloads
Published
Issue
Section
Categories
License
Copyright (c) 2023 Qiangqiang Zhou, Xiaoya Liu, Huifang Yun, Yahong Zhao, Kun Shu, Yong Chen, Song Chen

This work is licensed under a Creative Commons Attribution 4.0 International License.