Six RNA binding proteins (RBPs) related prognostic model predicts overall survival for clear cell renal cell carcinoma and is associated with immune infiltration
DOI:
https://doi.org/10.17305/bjbms.2021.6097Keywords:
RNA binding proteins, overall survival, clear cell renal cell carcinoma, model, prognosisAbstract
The aim of this article was to construct an accurate prognostic model by using RNA-binding proteins (RBPs) to predict overall survival (OS) for patients with clear cell renal cell carcinoma (ccRCC) as well as to reveal its associations with immune infiltration. Expression profiles based on RNA-binding proteins (RBPs) and clinical follow-up parameters were obtained from the Cancer Genome Atlas (TCGA) and the ArrayExpress databases. Through univariate COX and LASSO regression analyses, the RBPs based signature was developed. A total of six RBPs (CLK2, IGF2BP2, RNASE2, EZH2, PABPC1L, RPL22L1) were eventually used to establish a prognostic signature. Based on this signature, ccRCC patients were classified into high-risk and low-risk subgroups and significant OS was obtained in both the internal and external datasets (p<0.05). AUCs of its ROC curve were all above 0.70 and this signature was an independent prognostic factor of OS for ccRCC (p<0.05). Nomograms were also constructed to visualize the relationships among individual predictors and 1-, 3- and 5-year OS for ccRCC. Furthermore, the established RBPs based signature was strongly related to critical clinicopathologic characteristics such as grade (p=8.921e−12), stage (p=1.421e−11), M (p=1.662e−05), and T stage (p=7.907e−10). Moreover, 12 kinds of tumor-infiltrating immune cells were significantly linked to high-risk and low-risk groups classified by our constructed model (all p<0.05). Our study successfully identified six RBPs as a robust prognostic signature in ccRCC by both external and internal verification. Besides, our established model displayed significant associations with immune infiltration. In addition to original clinical parameters, our findings may further help clinicians in predicting patients’ survival status and creating individualized treatment plans.
Citations
Downloads
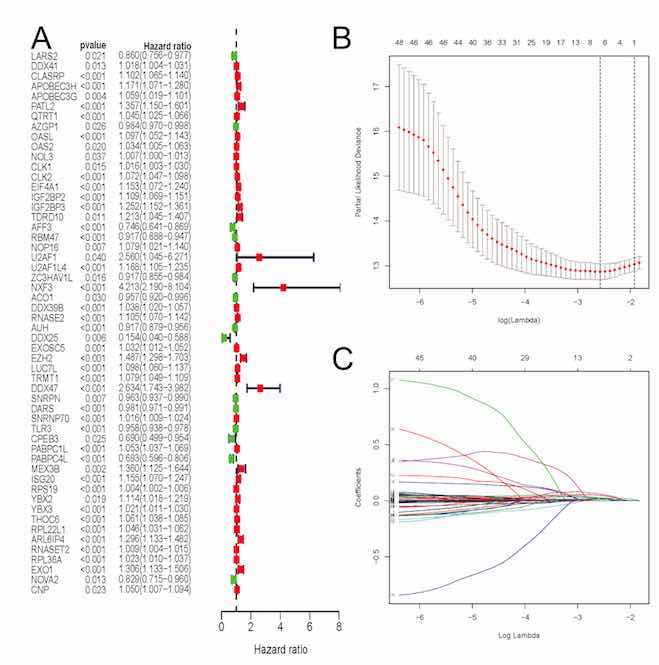
Downloads
Additional Files
Published
Issue
Section
Categories
License
Copyright (c) 2021 Qianwei Xing, Jiaochen Luan, Shouyong Liu, Limin Ma, Yi Wang

This work is licensed under a Creative Commons Attribution 4.0 International License.
How to Cite
Accepted 2021-08-17
Published 2022-06-01